
The same goes for line() and multi_line(), which you can use to make line graphs. You take the figure that you have created in the second step and by applying circle() or square() methods, you make sure that the data points that you want to scatter as circles and squares on your plot. You can not really keep up with all the glyphs that Bokeh has readily available for you, so the cheat sheet just lists the most important ones: scatter markers and line glyphs. When you have made a figure to plot in, the real work starts: adding renderers for your data and visual customizations, if necessary. You can, of course, also opt not to pass any arguments and just create a figure as is. You can use this function to create a new figure for plotting When you do create the new figure, there are some extra arguments that you can specify to make sure that you modify the figure according to your preferences: with plot_width and plot_height, you adjust the width and height of the plot, with the tools argument, you add certain tools to your plot, such as pan or box_zoom.Īlso, arguments such as x_range or y_range to set the range of your x-and y-axes can come in handy. To do this, you first need to import figure from otting. Much like when you’re working with Matplotlib, you start plotting with with Bokeh by initializing a figure.
Bokeh python how to#
If you’re unsure on how to create and work with DataFrames and arrays, consider taking DataCamp’s Pandas tutorial and NumPy tutorial, but also the Pandas cheat sheet and NumPy cheat sheet. However, you can also choose to create ColumnDataSource objects directly from dictionaries and Pandas DataFrames with ColumnDataSource() function, which you can use after importing it from bokeh.models. Under the hood, however, all data is converted to ColumnDataSource objects. You can pass data of all sorts, such as Python lists or tuples, NumPy arrays or Pandas DataFrames to make your plots. Seems simple, right? Let’s look at all these steps in more detail. You furthermore specify where you want to generate the output and then you show or save the results, as shown in the picture on the left-hand side. The basic steps that you need to take to make plots are five in total: you need your data to create new plots, to which you can add your glyphs and other visual customizations. Now, when you work with otting, you’ll see that there are two main components that you know to work efficiently with this interface: data and glyphs, which make up your plot. There’s also the bokeh.models interface that provides the most flexibility to application developers, but which isn’t included into this Bokeh cheat sheet. The Bokeh package offers a lot of flexibility to its users to make visualizations: the ones that are often used to quickly make plots and statistical charts are Bokeh’s mid-level general purpose otting interface and the high-level bokeh.charts interface.
Bokeh python for free#
That’s why DataCamp has collaborated with Bryan Van de Ven, Bokeh core contributor, on a Interactive Data Visualization with Bokeh course, which was recently launched and which guides you through the possibilities that this package has to offer step-by-step and in an interactive way.Īdditionally, DataCamp also made sure that you can download a Bokeh cheat sheet for free so that you have a handy reference sheet to fall back on when you’re in doubt! Bokeh Cheat Sheet The package offers lots of possibilities to visualize your data in a compelling way, but it’s also so flexible and big that once you want to get started, you can feel a bit overwhelmed by the possibilities. That’s where the Bokeh package comes in: the Python data visualization library that enables high-performance visual presentation of large datasets in modern web browsers. When you do start with taking these topics into account, you’ll mostly hear matplotlib is one of the preferred packages for data visualization and it is, but sometimes you do need to take your skills up a notch if you’re working with large datasets that you want to visualize interactively in web browsers. Conversely, this might also be one of the hardest steps in your data science learning, as visualizing data or to tell a story about your data in such a way that your information sees the information that your analysis brings to the table can be particularly challenging.
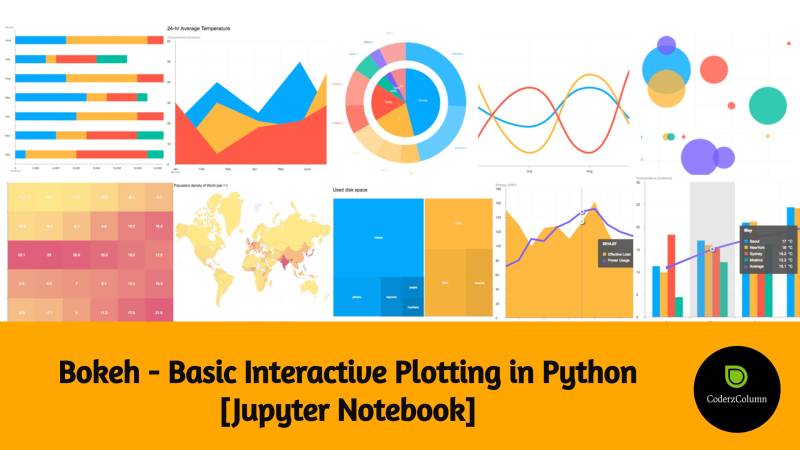

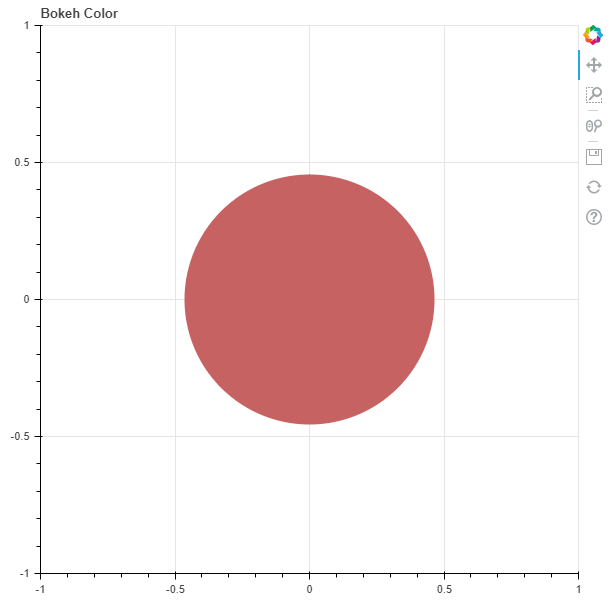
Python Data Visualization with Bokehĭata visualization and storytelling one of the steps in the data science workflow that are often forgotten. By Karlijn Willems, Data Science Journalist & DataCamp Contributor.
